Block Trade
A block trade is a high-volume transaction in a security that is privately negotiated and executed outside of the open market for that security. Major broker-dealers often provide "block trading" services—sometimes known as "upstairs trading desks"—to their institutional clients.In the United States and Canada a block trade is usually at least 10,000 shares of a stock or $100,000 of bonds but in practice significantly larger.
For instance, a hedge fund holds a large position in Company X and would like to sell it completely. If this were put into the market as a large sell order, the price would sharply drop. By definition, the stake was large enough to affect supply and demand causing a market impact. Instead, the fund may arrange for a block trade with another company through an investment bank, benefiting both parties: the selling fund gets a more attractive purchase price, while the purchasing company can negotiate a discount off the market rates. Unlike large public offerings, for which it often takes months to prepare the necessary documentation, block trades are usually carried out at short notice and closed quickly.
For a variety of reasons, block trades can be more difficult than other trades and often expose the broker-dealer to more risk. Most notably, because the broker-dealer is committing to a price for a large amount of securities, any adverse market movement can saddle the broker-dealer with a large loss if the position has not been sold. As such, engaging in block trading can tie up a broker-dealer's capital. Further, the fact that a large, well-informed money manager wants to sell (or perhaps buy) a large position in a particular security may connote future price movements (i.e., the money manager may have an informational advantage); by taking the opposite side of the transaction, the broker-dealer runs the risk of "adverse selection".
Block trading is a useful measure for analysts in order to assess where institutional investors are pricing a stock, because in a merger or acquisition, a bid needs to "clear the market" (i.e. enough shareholders need to tender), it is most useful to see at what prices large blocks of stock are trading. These prices imply what the largest shareholders are willing to sell their shares for; therefore, in block trading analysis, small trades are ignored to avoid skewing the data.
Program trading is a type of trading in securities, usually consisting of baskets of fifteen stocks or more that are executed by a computer program simultaneously based on predetermined conditions. Program trading is often used by hedge funds and other institutional investors pursuing index arbitrage or other arbitrage strategies. There are essentially two reasons to use program trading, either because of the desire to trade many stocks simultaneously (for example, when a mutual fund receives an influx of money it will use that money to increase its holdings in the multiple stocks which the fund is based on), or alternatively to arbitrage temporary price discrepancies between related financial instruments, such as between an index and its constituent parts.
Program trading
According to the New York Stock Exchange, in 2006 program trading accounts for about 30% and as high as 46.4% of the trading volume on that exchange every day. Barrons breaks down its weekly figures for program trading between index arbitrage and other types of program trading. As of July 2012, program trading made up about 25% of the volume on the NYSE; index arbitrage made up less than 1%.
History
Several factors help to explain the explosion in program trading. Technological advances spawned the growth of electronic communication networks. These electronic exchanges, like Instinet and Archipelago Exchange, allow thousands of buy and sell orders to be matched very rapidly, without human intervention.
In addition, the proliferation of hedge funds with all their sophisticated trading strategies have helped drive program-trading volume.
As technology advanced and access to electronic exchanges became easier and faster, program trading developed into the much broader algorithmic trading and high-frequency trading strategies employed by the investment banks and hedge funds.
Program trading firms
Program Trading is a strategy normally used by large institutional traders. Barrons shows a detailed breakdown of the NYSE-published program trading figures each week, giving the figures for the largest program trading firms (such as investment banks).
Index arbitrage
Index Arbitrage is a particular type of Program Trading which attempts to profit from price discrepancies between the basket of stocks which make up a stock index and its derivatives (such as the future based on that index). As of July 2012, it makes up less than 5% of the active Program Trading volume on the NYSE daily.
Premium buy and sell execution levels
The "premium" (PREM) or "spread" is the difference between the stock index future fair value and the actual index level. As the derivative is based on the index, the two should normally have a very close relationship. If there is a sufficiently large difference the arbitraging program will attempt to buy the relatively cheap level (whether that is the basket of stocks which make up the index or the index future) and sell the relatively expensive product, making money from the price discrepancy. The fair value calculation takes into account the time to expiration of the future contract, the dividends received from holding all the stocks, and the interest cost of buying the stocks.
Automated trading system
An automated trading system (ATS), a subset of algorithmic trading, uses a computer program to create buy and sell orders and automatically submits the orders to a market center or exchange. The computer program will automatically generate orders based on predefined set of rules using a trading strategy which is based on technical analysis, advanced statistical and mathematical computations or input from other electronic sources.
Automated trading systems are often used with electronic trading in automated market centers, including electronic communication networks, "dark pools", and automated exchanges. Automated trading systems and electronic trading platforms can execute repetitive tasks at speeds orders of magnitude greater than any human equivalent. Traditional risk controls and safeguards that relied on human judgment are not appropriate for automated trading and this has caused issues such as the 2010 Flash Crash. New controls such as trading curbs or 'circuit breakers' have been put in place in some electronic markets to deal with automated trading systems.
Mechanism
The automated trading system determines whether an order should be submitted based on, for example, the current market price of an option and theoretical buy and sell prices. The theoretical buy and sell prices are derived from, among other things, the current market price of the security underlying the option. A look-up table stores a range of theoretical buy and sell prices for a given range of current market price of the underlying security. Accordingly, as the price of the underlying security changes, a new theoretical price may be indexed in the look-up table, thereby avoiding calculations that would otherwise slow automated trading decisions. A distributed processing on-line automated trading system uses structured messages to represent each stage in the negotiation between a market maker (quoter) and a potential buyer or seller (requestor).
Advantages of Automated Trading System:
Minimizes Emotion
As orders are processed automatically once the pre-set rules are satisfied, emotional mistakes are minimized. It also helps traders to stay disciplined when the market is highly volatile.
Ability to Backtest
Before actually using the automated trading or the underlying algorithm, traders are able to evaluate their rules using the old data. It allows the traders to minimize potential mistakes and determine the expected returns.
Achieves Consistency
As orders are processed only when the pre-set rules are satisfied and traders only trade by plan, it helps the traders achieve consistency.
Improved Order Entry Speed
As computers process the orders as soon as the pre-set rules are met, it achieves higher order entry speed which is extremely beneficial in the current market where market conditions can change very rapidly.
Diversifies Trading
Automated trading systems allow users to simultaneously trade in multiple accounts which allows them to diversify their portfolio. Diversifying the portfolio allows the users to minimize their risks by spreading the risk over various instruments.
Disadvantages of Automated Trading System:
Mechanical Failures
Even though the underlying algorithm is capable of performing well in the live market, an internet connection malfunction could lead to a failure.
Monitoring
Although the computer is processing the orders, it still needs to be monitored because it is susceptible to technology failures as shown above.[6]
Over-Optimization
An algorithm that performs very well on backtesting could end up performing very poorly in the live market. Good performance on backtesting could lead to overly optimistic expectations from the traders which could lead to big failures.
Strategies:
Trend following
"The most common strategy which is implemented by following the trend in moving averages, channel breakouts, price level movements, and related technical indicators".
For example, the following formula could be used for trend following strategy:
"Consider a complete probability space (Ω, F, P). Let {\displaystyle S_{r}}S_r denote the stock price at time {\displaystyle r}r satisfying the equation
{\displaystyle dS_{r}=S_{r}[\mu (\alpha {r})dr+\sigma dB{r}],}{\displaystyle dS_{r}=S_{r}[\mu (\alpha {r})dr+\sigma dB{r}],} {\displaystyle S_{t}=X,}{\displaystyle S_{t}=X,} {\displaystyle t\leq r\leq T<\infty }{\displaystyle t\leq r\leq T<\infty },
where {\displaystyle \alpha _{r}\in {1,2}}{\displaystyle \alpha _{r}\in {1,2}} is a two-state Markov-Chain, {\displaystyle \mu (i)\equiv \mu {i}}{\displaystyle \mu (i)\equiv \mu {i}} is the expected return rate in regime {\displaystyle i=1,2,\sigma >0}{\displaystyle i=1,2,\sigma >0} is the constant volatility, {\displaystyle B{r}}{\displaystyle B{r}} is a standard Brownian motion, and {\displaystyle t}t and {\displaystyle T}T are the initial and terminal times, respectively".
Volume-weighted average price:
"Volume weighted average price strategy breaks up a large order and releases dynamically determined smaller chunks of the order to the market using stock-specific historical volume profiles."
According to Volume-weighted average price Wikipedia page, VWAP is calculated using the following formula:
":{\displaystyle P_{\mathrm {VWAP} }={\frac {\sum {j}{P{j}\cdot Q_{j}}}{\sum {j}{Q{j}}}},}P_{{{\mathrm {VWAP}}}}={\frac {\sum {{j}}{P{j}\cdot Q_{j}}}{\sum {j}{Q{j}}}},
where:
{\displaystyle P_{\mathrm {VWAP} }}P_{{{\mathrm {VWAP}}}} is Volume Weighted Average Price;
{\displaystyle P_{j}}P_{j} is price of trade {\displaystyle j}j;
{\displaystyle Q_{j}}Q_{j} is quantity of trade {\displaystyle j}j;
{\displaystyle j}j is each individual trade that takes place over the defined period of time, excluding cross trades and basket cross trades".
Mean reversion (finance)
This strategy is based on the idea that the values/prices of assets will revert to their mean prices/values.
"A continuous mean-reverting time series can be represented by an Ornstein-Uhlenbeck stochastic differential equation:
{\displaystyle dx_{t}=\theta (\mu -x_{t})dt+\sigma dW_{t}}{\displaystyle dx_{t}=\theta (\mu -x_{t})dt+\sigma dW_{t}}
where:
{\displaystyle P_{\mathrm {VWAP} }}P_{{{\mathrm {VWAP}}}} is Volume Weighted Average Price;
{\displaystyle P_{j}}P_{j} is price of trade {\displaystyle j}j;
{\displaystyle Q_{j}}Q_{j} is quantity of trade {\displaystyle j}j;
{\displaystyle j}j is each individual trade that takes place over the defined period of time, excluding cross trades and basket cross trades".
Mean reversion (finance)
This strategy is based on the idea that the values/prices of assets will revert to their mean prices/values.
"A continuous mean-reverting time series can be represented by an Ornstein-Uhlenbeck stochastic differential equation:
{\displaystyle dx_{t}=\theta (\mu -x_{t})dt+\sigma dW_{t}}
Where {\displaystyle \theta }\theta is the rate of reversion to the mean, {\displaystyle \mu }\mu is the mean value of the process, {\displaystyle \sigma }\sigma is the variance of the process and {\displaystyle W_{t}}W_{t} is a Wiener Process or Brownian Motion".
History
The concept of automated trading system was first introduced by Richard Donchian in 1949 when he used a set of rules to buy and sell the funds. Then, in the 1980s, the concept of rule based trading became more popular when famous traders like John Henry began to use such strategies. In the mid 1990s, some models were available for purchase. Also, improvements in technology increased the accessibility for retail investors.The early form of an Automated Trading System, composed of software based on algorithms, that have historically been used by financial managers and brokers. This type of software was used to automatically manage clients' portfolios. However, the first service to free market without any supervision was first launched in 2008 which was Betterment by Jon Stein. Since then, this system has been improving with the development in the IT industry. Now, Automated Trading System is managing huge assets all around the globe. In 2014, more than 75 percent of the stock shares traded on United States exchanges (including the New York Stock Exchange and NASDAQ) originated from automated trading system orders.
Applications
How it Works
Automated trading system can be based on a predefined set of rules which determine when to enter an order, when to exit a position, and how much money to invest in each trading product. Trading strategies differ such that while some are designed to pick market tops and bottoms, others follow a trend, and others involve complex strategies including randomizing orders to make them less visible in the marketplace. ATSs allow a trader to execute orders much quicker and to manage their portfolio easily by automatically generating protective precautions.
Backtesting:
Backtesting of a trading system involves programmers running the program by using historical market data in order to determine whether the underlying algorithm can produce the expected results. Backtesting software enables a trading system designer to develop and test their trading systems by using historical market data and optimizing the results obtained with the historical data. Although backtesting of automated trading systems cannot accurately determine future results, an automated trading system can be backtested by using historical prices to see how the system would have performed theoretically if it had been active in a past market environment.
Forward Testing
Forward testing of an algorithm can also be achieved using simulated trading with real-time market data to help confirm the effectiveness of the trading strategy in the current market. It may be used to reveal issues inherent in the computer code.
Live Testing
Live testing is the final stage of the development cycle. In this stage, live performance is compared against the backtested and walk forward results. Metrics compared include Percent Profitable, Profit Factor, Maximum Drawdown and Average Gain per Trade. The goal of an automated trading system is to meet or exceed the backtested performance with a high efficiency rating.
Market disruption and manipulation:
Automated trading, or high-frequency trading, causes regulatory concerns as a contributor to market fragility. United States regulators have published releases discussing several types of risk controls that could be used to limit the extent of such disruptions, including financial and regulatory controls to prevent the entry of erroneous orders as a result of computer malfunction or human error, the breaching of various regulatory requirements, and exceeding a credit or capital limit.
The use of high-frequency trading (HFT) strategies has grown substantially over the past several years and drives a significant portion of activity on U.S. markets. Although many HFT strategies are legitimate, some are not and may be used for manipulative trading. A strategy would be illegitimate or even illegal if it causes deliberate disruption in the market or tries to manipulate it. Such strategies include "momentum ignition strategies": spoofing and layering where a market participant places a non-bona fide order on one side of the market (typically, but not always, above the offer or below the bid) in an attempt to bait other market participants to react to the non-bona fide order and then trade with another order on the other side of the market. They are also referred to as predatory/abusive strategies. Given the scale of the potential impact that these practices may have, the surveillance of abusive algorithms remains a high priority for regulators. The Financial Industry Regulatory Authority (FINRA) has reminded firms using HFT strategies and other trading algorithms of their obligation to be vigilant when testing these strategies pre- and post-launch to ensure that the strategies do not result in abusive trading.
FINRA also focuses on the entry of problematic HFT and algorithmic activity through sponsored participants who initiate their activity from outside of the United States. In this regard, FINRA reminds firms of their surveillance and control obligations under the SEC's Market Access Rule and Notice to Members 04-66, as well as potential issues related to treating such accounts as customer accounts, anti-money laundering, and margin levels as highlighted in Regulatory Notice 10-18 and the SEC's Office of Compliance Inspections and Examination's National Exam Risk Alert dated September 29, 2011.
FINRA conducts surveillance to identify cross-market and cross-product manipulation of the price of underlying equity securities. Such manipulations are done typically through abusive trading algorithms or strategies that close out pre-existing option positions at favorable prices or establish new option positions at advantageous prices.
In recent years, there have been a number of algorithmic trading malfunctions that caused substantial market disruptions. These raise concern about firms' ability to develop, implement, and effectively supervise their automated systems. FINRA has stated that it will assess whether firms' testing and controls related to algorithmic trading and other automated trading strategies are adequate in light of the U.S. Securities and Exchange Commission and firms' supervisory obligations. This assessment may take the form of examinations and targeted investigations. Firms will be required to address whether they conduct separate, independent, and robust pre-implementation testing of algorithms and trading systems. Also, whether the firm's legal, compliance, and operations staff are reviewing the design and development of the algorithms and trading systems for compliance with legal requirements will be investigated. FINRA will review whether a firm actively monitors and reviews algorithms and trading systems once they are placed into production systems and after they have been modified, including procedures and controls used to detect potential trading abuses such as wash sales, marking, layering, and momentum ignition strategies. Finally, firms will need to describe their approach to firm-wide disconnect or "kill" switches, as well as procedures for responding to catastrophic system malfunctions.[citation needed]
Notable examples
Examples of recent substantial market disruptions include the following:
On May 6, 2010, the Dow Jones Industrial Average declined about 1,000 points (about 9 percent) and recovered those losses within minutes. It was the second-largest point swing (1,010.14 points) and the largest one-day point decline (998.5 points) on an intraday basis in the Average's history. This market disruption became known as the Flash Crash and resulted in U.S. regulators issuing new regulations to control market access achieved through automated trading.
On August 1, 2012, between 9:30 a.m. and 10:00 a.m. EDT, Knight Capital Group lost four times its 2011 net income. Knight's CEO Thomas Joyce stated, on the day after the market disruption, that the firm had "all hands on deck" to fix a bug in one of Knight's trading algorithms that submitted erroneous orders to exchanges for nearly 150 different stocks. Trading volumes soared in so many issues, that the SPDR S&P 500 ETF (SYMBOL: SPY), which is generally the most heavily traded U.S. security, became the 52nd-most traded stock on that day, according to Eric Hunsader, CEO of market data service Nanex. Knight shares closed down 62 percent as a result of the trading error and Knight Capital nearly collapsed. Knight ultimately reached an agreement to merge with Getco, a Chicago-based high-speed trading firm.
High-frequency trading
High-frequency trading (HFT) is a type of algorithmic financial trading characterized by high speeds, high turnover rates, and high order-to-trade ratios that leverages high-frequency financial data and electronic trading tools. While there is no single definition of HFT, among its key attributes are highly sophisticated algorithms, co-location, and very short-term investment horizons. HFT can be viewed as a primary form of algorithmic trading in finance. Specifically, it is the use of sophisticated technological tools and computer algorithms to rapidly trade securities. HFT uses proprietary trading strategies carried out by computers to move in and out of positions in seconds or fractions of a second.
In 2017, Aldridge and Krawciw estimated that in 2016 HFT on average initiated 10–40% of trading volume in equities, and 10–15% of volume in foreign exchange and commodities. Intraday, however, the proportion of HFT may vary from 0% to 100% of short-term trading volume. Previous estimates reporting that HFT accounted for 60–73% of all US equity trading volume, with that number falling to approximately 50% in 2012 were highly inaccurate speculative guesses. High-frequency traders move in and out of short-term positions at high volumes and high speeds aiming to capture sometimes a fraction of a cent in profit on every trade. HFT firms do not consume significant amounts of capital, accumulate positions or hold their portfolios overnight. As a result, HFT has a potential Sharpe ratio (a measure of reward to risk) tens of times higher than traditional buy-and-hold strategies. High-frequency traders typically compete against other HFTs, rather than long-term investors. HFT firms make up the low margins with incredibly high volumes of trades, frequently numbering in the millions.
A substantial body of research argues that HFT and electronic trading pose new types of challenges to the financial system. Algorithmic and high-frequency traders were both found to have contributed to volatility in the Flash Crash of May 6, 2010, when high-frequency liquidity providers rapidly withdrew from the market. Several European countries have proposed curtailing or banning HFT due to concerns about volatility.
History
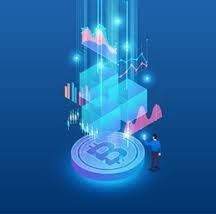
High-frequency trading has taken place at least since the 1930s, mostly in the form of specialists and pit traders buying and selling positions at the physical location of the exchange, with high-speed telegraph service to other exchanges.
The rapid-fire computer-based HFT developed gradually since 1983 after NASDAQ introduced a purely electronic form of trading. At the turn of the 21st century, HFT trades had an execution time of several seconds, whereas by 2010 this had decreased to milli- and even microseconds. Until recently, high-frequency trading was a little-known topic outside the financial sector, with an article published by the New York Times in July 2009 being one of the first to bring the subject to the public's attention.
On September 2, 2013, Italy became the world's first country to introduce a tax specifically targeted at HFT, charging a levy of 0.02% on equity transactions lasting less than 0.5 seconds.
Market growth
In the early 2000s, high-frequency trading still accounted for fewer than 10% of equity orders, but this proportion was soon to begin rapid growth. According to data from the NYSE, trading volume grew by about 164% between 2005 and 2009 for which high-frequency trading might be accounted. As of the first quarter in 2009, total assets under management for hedge funds with high-frequency trading strategies were $141 billion, down about 21% from their peak before the worst of the crises, although most of the largest HFTs are actually LLCs owned by a small number of investors. The high-frequency strategy was first made popular by Renaissance Technologies who use both HFT and quantitative aspects in their trading. Many high-frequency firms are market makers and provide liquidity to the market which lowers volatility and helps narrow bid–offer spreads, making trading and investing cheaper for other market participants.
Market share
In the United States in 2009, high-frequency trading firms represented 2% of the approximately 20,000 firms operating today, but accounted for 73% of all equity orders volume.[citation needed] The major U.S. high-frequency trading firms include Virtu Financial, Tower Research Capital, IMC, Tradebot and Citadel LLC. The Bank of England estimates similar percentages for the 2010 UK market share, also suggesting that in Europe HFT accounts for about 40% of equity orders volume and for Asia about 5–10%, with potential for rapid growth. By value, HFT was estimated in 2010 by consultancy Tabb Group to make up 56% of equity trades in the US and 38% in Europe.
As HFT strategies become more widely used, it can be more difficult to deploy them profitably. According to an estimate from Frederi Viens of Purdue University, profits from HFT in the U.S. has been declining from an estimated peak of $5bn in 2009, to about $1.25bn in 2012.
Though the percentage of volume attributed to HFT has fallen in the equity markets, it has remained prevalent in the futures markets. According to a study in 2010 by Aite Group, about a quarter of major global futures volume came from professional high-frequency traders. In 2012, according to a study by the TABB Group, HFT accounted for more than 60 percent of all futures market volume in 2012 on U.S. exchanges.
Do vote @Blurt.Live as your Witness
Posted from https://blurt.live